Noah Brier | February 22, 2023
The Synthetic Research Edition
On AI, surveys, and using large language models for research
Hi all, I ran this over at my marketing AI newsletter today and thought it would make an interesting WITI. I hope you enjoy and if you want more of this kind of content, subscribe to BrXnd Dispatch. - Noah (NRB)
Noah here. A few weeks ago, I heard the term “silicon sampling” for the first time. As Paul Aaron laid out in an excellent piece for the Addition newsletter, the basic idea is that GPT3 and similar large language models can simulate the opinions of real people for polling and research purposes. The September 2022 paper “Out of One, Many: Using Language Models to Simulate Human Samples” lays out evidence that the biases of these models can actually be utilized as a feature to generate synthetic citizens for research purposes.
Want to run a WITI classified? Want to reach 20,000 brilliant readers? WITI classifieds start at $250, and you can purchase one through this form. If you buy this week, we’ll throw an extra week in for free on any ad. If you have any questions, don’t hesitate to drop us a line.
The paper’s authors refer to this capability as “algorithmic fidelity”—easier understood as the ability to induce the model to produce answers that correlate with the opinions of specific groups:
Our conception of algorithmic fidelity goes beyond prior observations that language models reflect human-like biases present in the text corpora used to create them. Instead, it suggests that the high-level, human-like output of language models stems from human-like underlying concept associations. This means that given basic human demographic background information, the model exhibits underlying patterns between concepts, ideas, and attitudes that mirror those recorded from humans with matching backgrounds. To use terms common to social science research, algorithmic fidelity helps to establish the generalizability of language models, or the degree to which we can apply what we learn from language models to the world beyond those models.
They went on to test this fidelity across several criteria, including how well it could recreate survey results.
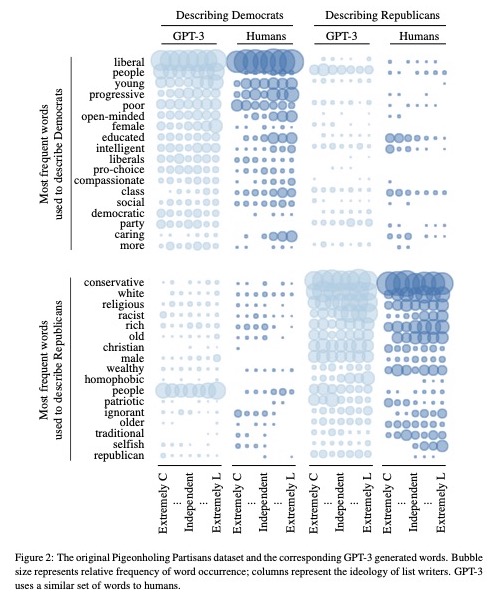
While this paper focuses mainly on political polling, there are fascinating implications for marketing and brand research (as Paul also points out in his article). Brands spend millions of dollars annually studying consumer perception, product opportunities, and advertising messaging. If a large language model could produce even a small amount of this research, it would save companies huge amounts of time and money.
Why is this interesting?
To learn more about the approach and its application, I reached out to Hugo Alves, the co-founder of the newly-launched company Synthetic Users, which practices this “silicon sampling” approach for product research. The idea for Synthetic Users came to Alves when he heard about the Google engineer that claimed the company’s language model LaMDA was sentient. If these models were convincing enough to trick engineers, Alves thought, then surely they could be used for product research. When he came across a few papers on the topic, including the research cited above, he decided to focus his energy on commercializing the approach. “You define your audience, you define what you feel the problems are, and you define your proposed solution,” said Alves, explaining how the Synthetic Users product works today. “Then the system generates up to 10 interviews each time you run it. It also generates a report from those interviews. So essentially, it’s an easy, almost real-time, way to get feedback on your ideas and propositions.”
It’s in its early days, but the plan is to continue to simplify the product, requiring less direct knowledge of the solution. “In the future,” Alves explained, “we want this to be even richer, so you just define your audience like, ‘people in long-distance relationships,’ and you click a button, and it gives you a set of possible problems they have, and you can select from them instead of you having to go and put it in yourself.” I asked Hugo if he had plans to expand the target audience to marketers, and he said they’re still in the early stages of the product lifecycle but are leaving all avenues open.
One question all this raises for me is, “why?” If it is so easy to reproduce research from human panels, on the one hand, it makes tons of sense to do it cheaper and quicker using machine learning models. Still, on the other, it makes you wonder if that kind of research is actually worth anything in the first place. Anyone who has worked with large brands on research has seen all sorts of output—a ton of marketing research exists to ensure a marketing VP or agency has something to point to if everything goes wrong. Synthetic research seems like a perfect solution for this kind of study, where the goal is clearly not to gain deep insight into a target audience. When I posed this to Alves, who has a master’s in clinical psychology, he said, “One of the things I say to people who ask, ‘but does a language model have all the nuances and subtleties?’ And I’m like, ‘have you seen real research? Have you seen real user researchers doing it? And the feedback that sometimes you get it?’ Even with just our beta product, it’s comparable.”
That makes sense to me, at least in theory. I’m supposed to get access to the Synthetic Users product this week, and I am excited to have a play and share the results. (NRB)
—
Thanks for reading,
Noah (NRB) & Colin (CJN)
—
Why is this interesting? is a daily email from Noah Brier & Colin Nagy (and friends!) about interesting things. If you’ve enjoyed this edition, please consider forwarding it to a friend. If you’re reading it for the first time, consider subscribing.